By Sara Loo
You don’t need me to tell you that things are not as they used to be. Unless you have recently emerged from a 3-month long hike, you should be well aware of the current global atmosphere. Working from home, social distancing, lockdown. Words that have entered our everyday vernacular. There is a never-ending stream of news and updated case numbers, late night press conferences and a continually changing list of do’s and don’ts.
Staying informed is important. But in these anxious times, keeping up to date can be exhausting. I have seen more scientific terms in the news than ever, and have been sent articles from family and friends with words I thought were confined to the depths of my research, never to see the light of day.
To non-mathematicians, there is a long list of scientific and mathematical terms to be understood, and just So. Much. News. COVID-19 has taken over the news cycle. And, with it come phrases like enveloped viruses, antivirals, doubling time, mortality and morbidity, exponential growth.
Though I do not by any means claim to be an expert in viral epidemiology, I currently work in understanding the evolution of infectious diseases and can claim to knowing something or other about mathematics. And there may be no better time than now to spread the good news of mathematics and its usefulness to our everyday lives.
Exponential growth
An exponential graph can be scary to look at. When we’re tracking cases of a disease and seeing it get larger, and then get larger even quicker, it’s easy to see how the changing numbers may fan our fear.
However, it’s important to note that exponential growth has its limits, and can be slowed by employing certain preventative measures, as we’ve seen in countries such as Singapore, Japan and South Korea – countries that seem to have successfully ‘flattened the curve’.
Even in the most dire case, the curve will flatten when everyone has become infected; when there’s no one else left to infect.
As an example of exponential growth slowing down, let’s take for example your productivity during a day of working from home. You might start the day with the goal of writing a scientific manuscript, or a blog piece. It might be slow to get going. Other than the inevitable writer’s block, there’s that second coffee you need to go to the kitchen to make, and a child’s IT issues to fix so they can join their online class.
Once you have that hot cuppa and you’ve told your child to “try turning it off and on again”, you get to sit down and focus. Then, the writing just starts. Words flow quickly.
But then you have lunch, and you accidentally eat too much of last night’s leftover pasta. Your kids get more and more irritable as they get bored of being cooped up in front of their computer screen, starting to throw said leftover pasta at each other. Things get crazy at home.
Your manuscript sits forgotten for a time. Nothing new is written. After returning to your desk, you start typing, but slowly. The excess carbs slow your thinking. The length of your article still grows, but slowly.
Like the speed of your writing in a day of working from home, we want to slow down the spread of infection. We want to impose a post-lunch carbo crash onto the outbreak. We want to reduce new cases quickly.
SIR modelling
You may have seen this simulation of little dots getting infected with COVID-19, and the effects of social distancing on the overall spread of the outbreak (if you haven’t, please do. It’s a great visualisation). This is based on a simple mathematical model commonly used in epidemiology known as an SIR model.
The letters stand for Susceptible, Infectious, and Recovered. Individuals can be susceptible to a disease, be infected by a disease, or have recovered from that disease.
Mathematical modelling is based around tracking changes to different categories of things (in this case, categories of people and their state based on whether they have a certain disease or not), assuming these changes follow a set of average behaviours. An SIR model follows this basic structure.
Based on what we know about these average behaviours, how will the outbreak progress?
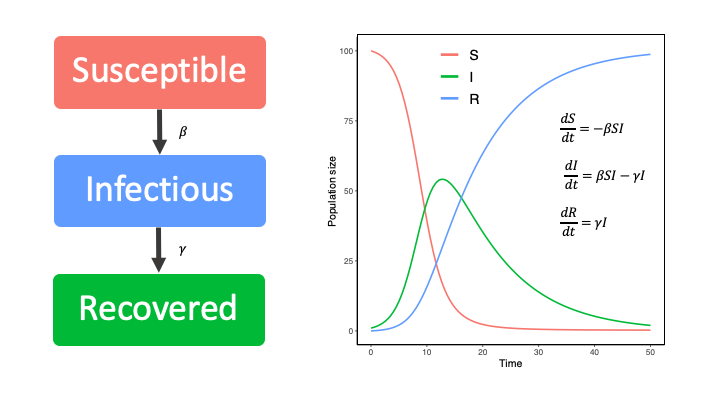
If 1 infected individual, say, hops off a plane into Sydney Airport, will an outbreak in Sydney? If there are 100 people in the arrival hall of the airport at the time they arrive, and we know the disease is transmissible at a certain rate, will there be an outbreak in Sydney?
Will all the susceptible individuals become infectious, provided they move from susceptible to infectious at a certain rate (β)?
R0
Understanding how quickly the outbreak progresses in infectious disease modelling revolves around a value called R0 (pron. arr-naught). The basic description of R0 is that it is a measure of the average number of new infections every infected individual is likely to cause.
Without going into the mathematical details, this is often dependent on the population size, the rate of transmission (how easy it is to transmit – i.e. can I get it by just touching the same elevator button as someone who is sick, or do they need to sneeze directly into my mouth), and how many other people are around that are likely to get sick too.
Numerically, an R0 greater than 1 indicates that a disease will spread throughout a population. Public health initiatives aim to reduce this value to less than 1, in order to minimise the effect of a disease outbreak and eliminate it from a population.
#stayhome aims to reduce this R0 by effectively removing people from the population. If they’re infected they can’t infect any more people. If they’re susceptible, they can’t get infected and subsequently infect more people.
You may have seen this represented in the form of match sticks, dominoes, or a family-tree-like transmission chain. Whatever the medium you’ve seen the information conveyed, the message is the same. If possible, stay home. It may not be possible for everyone, but for those who can: stay home.
While we are living in uncertain times, and though mathematics has the reputation of being stressful and equally anxiety-inducing, the numbers and predictions you see in the news are based on well-studied models. I, for one, take comfort in that.